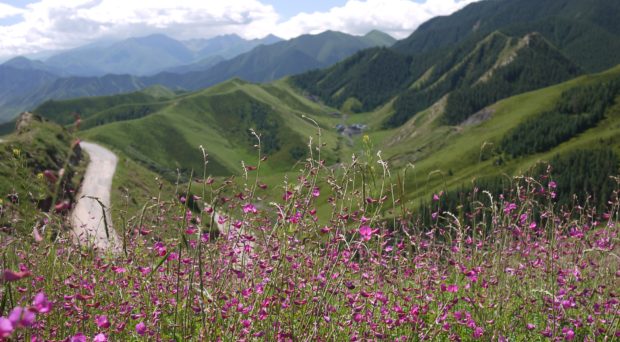
Human echinococcosis – distribution and impact
Echinococcosis is a globally distributed zoonotic disease caused by infection with larval stages of parasitic tapeworms from the genus Echinoccocus.
Current estimates by the W.H.O suggest that globally, more than 1 million people are infected with echinococcosis, leading to approximately 871 000 disability-adjusted life-years (DALYs) each year. As well as the substantial disease burden and deaths associated with human echinococcosis, treatment of livestock infections are estimated to be over $3 billion (USD) annually.
Treatment regimes are currently complicated and dangerous, and often require prolonged drug therapy and/or invasive surgery.
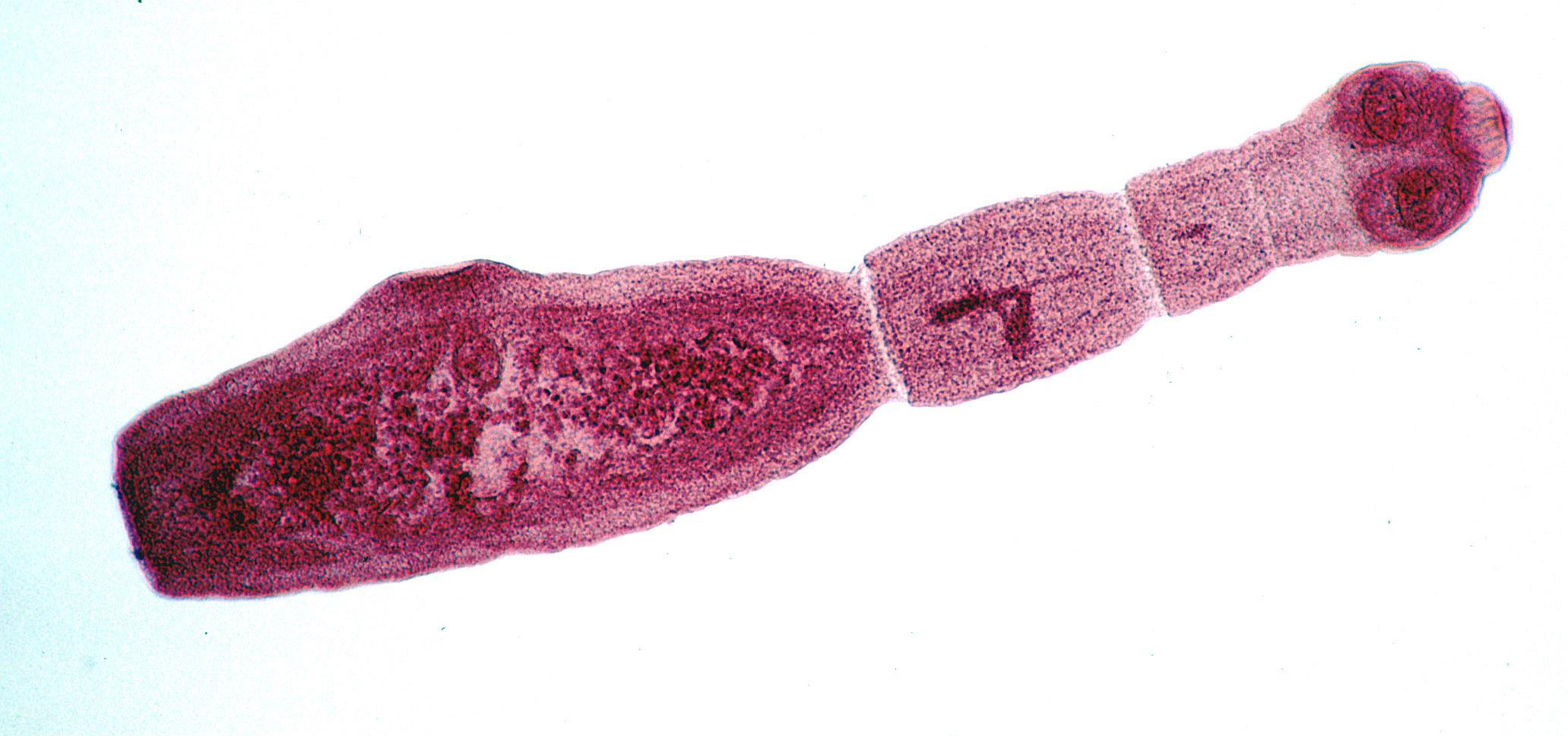
Of all the regions affected by human echinococcosis, Western China has the highest prevalence anywhere in the world, and as such the impact on public health in this region of China is huge, especially in rural, pastoral and economically disadvantaged areas.
The high endemicity of human echinococcosis in Western China has led researchers to investigate the geographical and environmental conditions of the region, and question whether specific environmental factors might influence transmission of echinococcosis to humans. For example, one study linked the prevalence of echinococcosis with annual mean precipitation, and another found a correlation between higher altitudes and increased echinococcosis prevalence.
Despite extensive work connecting specific environmental factors to human echinococcosis, as of yet no studies have researched how, or if, natural environmental factors can be predictors of human echinococcosis risk.
A recent study by Jie Yin and colleagues from the College of Global Change and Earth System Science in Beijing has set out to do just that, and are the first to model and predict the spatial distribution of human echinococcosis in Western China.
Creating and informing the model
To investigate the spatial distribution and environmental risk factors of human echinococcosis, researchers first selected 344 counties across Western China (see study map below) for inclusion in the study, and retrieved county-level prevalence data of human echinococcosis from local CDC reports and epidemiological studies (surveyed) for later comparison against predictions of prevalence made by their model.
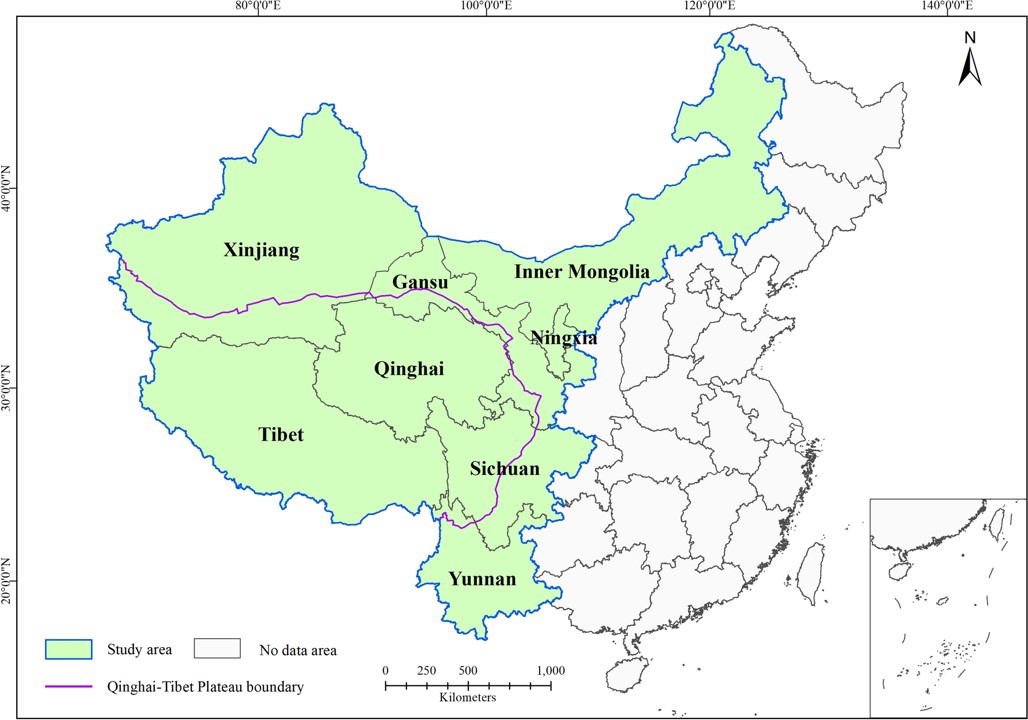
Nine environmental factors considered as natural risk factors for echinococcosis and four seasonal indices (spring, summer, autumn and winter) were chosen for the prediction, which fall into two categories, climate and geographical:
Climate factors:
- Temperature (T)
- Precipitation (Pre)
- Relative humidity (rh)
- Sunshine duration (sun)
Geographical factors:
- Elevation (measured as digital elevation model (DEM))
- Vegetation density (measured as the normalized difference vegetation index (NDVI))
- Grassland area ratio (GrassR)
- Forest area ratio (ForestR)
- Cultivated land area ratio (CultivatedR)
In order to analyse the relationship between the nine environmental factors and prevalence of echinococcosis in each county, and predict potential hot/cold spots of human prevalence, the researchers constructed a structured additive regression (STAR) mathematical model using Bayesian inference.
Insights into human echinococcus risk provided by the model
The predicted prevalence and hot/cold spot model for each county was generally in agreement with the prevalence and hot/cold spots reported by the surveyed findings, providing support for the constructed model.
The authors do note however, that modelled prevalence based on the environmental factors was in some instances higher than surveyed prevalence, suggesting that past control measures implemented in Western China have been effective in reducing human echinococcosis prevalence from what is possible based on the environmental conditions.
Highly endemic areas where prevalence is highest (>2%) were almost exclusively concentrated in the Qinghai-Tibet Plateau in South/Southwestern China. Counties outside the Plateau region generally had low predicted echinococcosis prevalence (<0.5%).
The three identified hot spots were thus also predicted to be located on the high altitude Qinghai-Tibet Plateau, indicating that environmental conditions are well suited for human echinococcosis transmission and that prevention and control should be focused in this region.
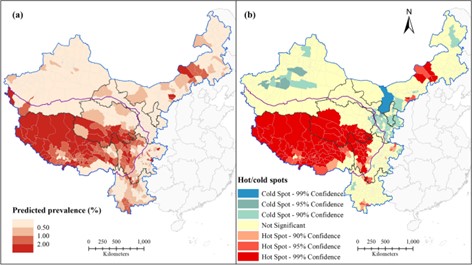
Key environmental factors influencing echinococcosis transmission
The model also revealed that both climate and geographical landscape factors had a significant impact on human echinococcosis prevalence in the region.
In particular, elevation (DEM), vegetation density (NDVI) in Spring, Summer precipitation (pre) and sunshine duration (sun), relative humidity (rh) in the Winter, GrassR, and ForestR were shown to be key environmental factors.
These factors likely influence the survival, infectivity, and successful release of Echinococcus eggs into the environment, and act as drivers of parasite transmission in Western China.
Conclusion
This work by Jie Yin and collueages illustrates how spatial epidemiological modelling is useful for informing real world disease prevention and control strategies, and that it can help improve prioritisation of prevention and control measures and lead to better allocation of resources to where they are needed most.
The authors note that additional risk factors of human echinococcosis, such as socioeconomic circumstances, population demographics, human behaviour, habitat type and animal infection status, could further improve echinococcosis prevalence predictions made by the model and enable its use in predicting hot spots for similar Neglected Tropical Diseases.
Comments