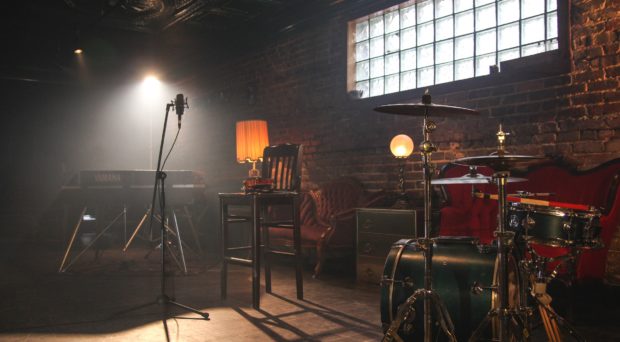
Popularity Bias in Music Recommendations
As more and more music is available via music streaming services, music recommendation systems have become essential for helping users to search, sort and filter extensive music collections.
Beyond-mainstream listeners receive worse music recommendations than mainstream listeners
However, it is a widely-known problem that recommender systems are prone to popularity bias, which leads to long-tail items (i.e., items with few user interactions) having little chance of being recommended.
We validated this effect by measuring the accuracy of algorithm-generated music recommendations for listeners of mainstream and beyond-mainstream music.
We used a dataset containing the listening histories of 4,148 users (2,074 users in each group) of the music streaming platform Last.fm who listened mostly to beyond-mainstream music (BeyMS) or mostly to mainstream music (MS).
Figure 1 shows that beyond-mainstream listeners receive worse music recommendations than mainstream listeners.

Subgroups of Beyond-Mainstream Music Listeners
We applied the unsupervised clustering algorithm HDBSCAN* to identify subgroups within the beyond-mainstream music listeners.
We identified four subgroups, which we labeled according to the types of music they most frequently listened to: (i) users of music genres with only acoustic instruments such as folk (U_folk), (ii) users of high-energy music such as hard rock or hip-hop (U_hard), (iii) users of music with acoustic instruments and (nearly) no vocals such as ambient (U_ambi), and (iv) users of high-energy music with (nearly) no vocals such as electronica (U_elec).
Impact on Music Recommendations
the willingness of users to listen to music outside their own music preferences has a positive effect on the quality of music recommendations
By comparing each subgroup’s listening histories, we identified users who were most likely to listen to music outside their preferred genres.
Those who mainly listened to acoustic music with (nearly) no vocals such as ambient (U_ambi) were found to be most likely to listen to music preferred by the other subgroups as well.
Those who mainly listened to high-energy music such as hard rock or hip-hop (U_hard) were least likely to listen to music preferred by the other subgroups.
In figure 2 below we see that U_ambi receives better recommendations than U_hard, which means that the willingness of users to listen to music outside their own main music preferences has a positive effect on the quality of music recommendations.
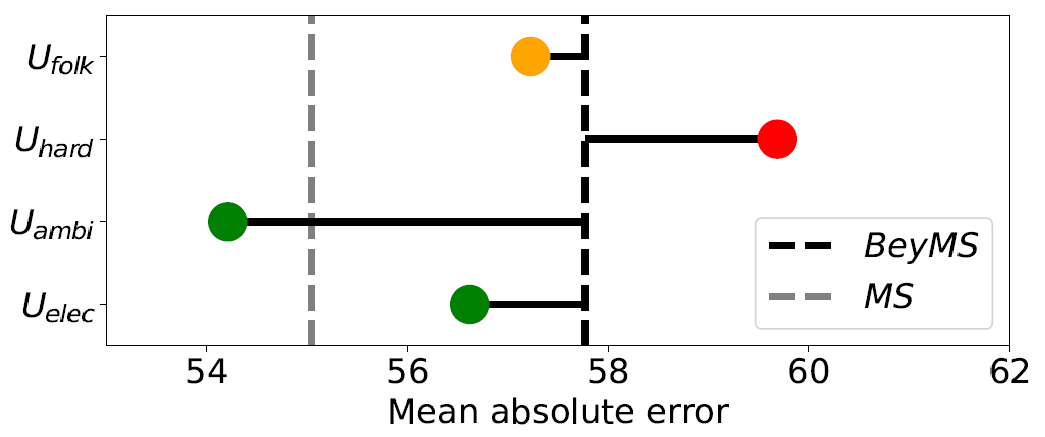
Towards Fair Music Recommendations
We believe that our findings provide valuable insights for developing improved user models and recommendation approaches to better serve beyond-mainstream music listeners.
However, we also believe that a lot of research is still needed to provide fair music recommendation models that are generalizable and avoid the unfair treatment of any user group.
We hope that our data set (https://doi.org/10.5281/zenodo.3784764) and source code (https://github.com/pmuellner/supporttheunderground) provided with the article are of use to the scientific community for future analyses.
Comments