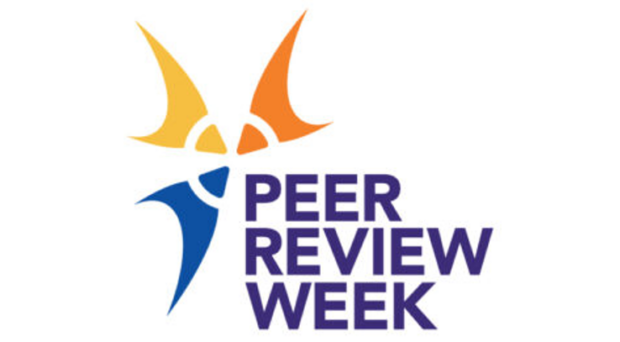
“AI will enhance the future of peer review by using automated systems to analyze and evaluate research articles. It will expedite the review process, improve accuracy, identify biases, and help in handling large volumes of submissions. However, human expertise and judgment will remain crucial in assessing the overall quality and impact of research.”
– ChatGPT-3.5
For many of us, conversations surrounding artificial intelligence (AI) and large language models (LLMs) seem inescapable. For some others, the thought of “let me ask ChatGPT about this” has become routine. Seemingly, AI has filtered into our everyday lives, a scientific innovation with an indiscriminate impact on the way we conduct scientific research. Natural Language Processing (NLP) is a branch of AI that deals with language processing and generation. Under this hierarchy, LLMs are a specific subset of NLP, which draws upon huge training datasets (of typically a billion parameters or more) to generate text, summarize and answer questions. Examples of commonly known LLMs include OpenAI’s ChatGPT-3.5 or -4, Microsoft’s Bing Chat and Google’s Bard.
As described in a recent Nature review, we are already seeing the multifaceted ways AI can be used to aid scientific discovery. From generating hypotheses to designing experiments, we have barely scratched the surface of harnessing this versatile tool. While much of the discussion has covered the potential benefits AI may bring, we are increasingly seeing concerns about the risks AI may carry. Echoing the plot of a dystopian sci-fi, some news outlets have gone so far as to state that this technology may pose an existential threat to humankind. Parallel with the rising use of LLMs, scientific publishing and academia are not absent from this nervous discourse, with much of the discussion focused around the use of AI tools in generating paper publications and peer review reports.
Computer-assistance is not a novel concept in scientific publishing. Automated systems have long been integrated into the process to scan references, inspect manuscripts for plagiarism and check their compliance with journal policies. However, the capacity of AI to expand the volume and type of tasks that can be automated poses several risks that could disrupt the peer review system:
- LLM-generated ‘peer review’ reports are at risk of error: its ability to conflate different sources of information can lead to inaccurate and misleading information (LLM developers refer to this phenomenon as ‘hallucination’).
- Although a powerful tool for processing and summarizing facts about the world, as their output is limited to the training data they are given, LLMs are not yet able to generate original information: this means that the produced reports may not necessarily be as critical as a human reviewer would be and are very rarely as insightful. They will also be subject to any biases inherent in the data they are fed.
- Developers and creators of LLMs are often not very transparent about how their models use and retain the prompts they receive, raising concerns about intellectual property and confidentiality.
- At a time when many academics already feel inundated with invitations to review a manuscript, LLMs are at risk of being misused by reviewers to rapidly generate poor quality, unhelpful reviews, thereby diminishing the value of the peer review process.
Such concerns have led some publishers and funding agencies such as the NIH to ban the use of AI in the peer review process. While these measures are expected and perhaps advisable as we come to better understand how to work with LLMs, we should not ignore their potential to help peer reviewers:
- LLMs have the potential to support reviewers as writing assistants in assembling constructive and easily readable reports
- With more than 90% of indexed scientific articles being published in English, English-speaking researchers are at an advantage in science communication, and the use of LLMs could help democratize peer review by allowing researchers who are not fluent English speakers to communicate science in English more effectively.
- While the risks of bias in LLM persist, the potential biases, judgments and subjectivity inherent in manual reviewing can be minimized by using these tools.
- A significant amount of research requires multidisciplinary scrutiny. LLMs could accelerate the assessment of aspects of papers that require significant time, energy and expertise (such as statistical validity)
- As a complementary tool to one’s own work, LLMs could expand on the existing human reviewers’ report by providing additional insights and context.
Scientific peer review has long been considered the gold standard for ensuring the quality and credibility of research. A way of replicating the research-focused discussions between academics that formed the foundation for the critical yet open-minded approach that defines modern scientific discovery. The use of LLMs in the scientific review process may, to some, feel like the removal of the ‘peer’ in peer review and, as such, a threat to science in its entirety. However, if used as a tool to assist the peer review process, rather than as a standalone “robot” reviewer, LLMs could enhance both the quality and efficiency of manuscript assessment.
Reaching this goal will require a concerted effort from all parties involved in the peer review process. Reviewers who use LLMs to assist with manuscript assessment must take responsibility for the accuracy and value of the reports they submit. Authors can use LLMs to help improve their work but should avoid the trap of assuming these imperfect tools can instantly create a complete manuscript. In turn, publishers must ensure that accountability across the peer review process remains in the hands of experienced human editors. Transparency and honesty about LLMs will be vital in shaping the policies that will help usher in their use.
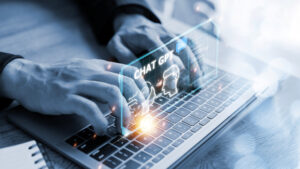
“AI can bring efficiency to peer review by identifying plagiarism, sorting submissions, and assessing the quality of research. However, it might also lead to job loss for human reviewers, lack of nuanced understanding, and the risk of overlooking innovative but unconventional studies. Additionally, reliance on AI may increase the risk of hacking and manipulation of review outcomes.”
– ChatGPT-4.0
The text at the top of the article was generated by ChatGPT3.5 in response to a prompt asking about the future of peer review. The text above, which provides a considerably more cautious perspective on AI, was created by the next iteration of ChatGPT. LLMs are a technology developing at an incredible pace, and opinions on its use are likely to shift just as rapidly. Science should strive to work with, rather than against or without, AI whilst recognizing the complexity of the challenges it proposes. Whatever these developments may bring, it is important that science maintains an attitude of critical open-mindedness and adaptability in the face of new tools and developments, like science always has.
The authors would like to acknowledge ChatGPT-3.5 and ChatGPT-4 for assistance in writing this piece.
Comments