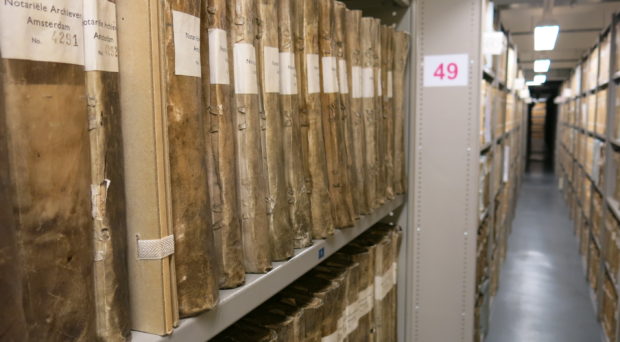
This post has been cross-posted from the SpringerOpen blog.
It possibly goes by unnoticed, but heritage institutions amass a large amount of data on the characteristics, condition and access of their collections. Some of this data is gathered automatically by collection management systems, and some can be purposely collected during regular surveys. The uncertainty of the resulting data – given the unknown past histories of real objects and their composition – requires robust methods of analysis; yet that data is a fundamental source of evidence for the causes of degradation of objects.
The uncertainty of the resulting data requires robust methods of analysis; yet that data is a fundamental source of evidence for the causes of degradation of objects.
Damage epidemiology for heritage ‘populations’
As we are dealing with defined populations (of objects), epidemiological studies offer the right methodology to explore the patterns of decay in subgroups of such populations, similar to epidemiological studies carried out in “living” populations. One of the study designs used in epidemiology are surveys. In the heritage field, surveys are a well-known tool to extract information from collections.
Surveys have so far mostly been used as descriptive cross-sectional studies to report the prevalence of certain types of damage. However, our approach to collection survey design shows us that surveys can also provide statistically significant information on the reasons for occurrence of the event of interest (e.g. object degradation), as long as data is purposely collected and statistically analysed.

Using datasets to inform preventive conservation decisions
In our research on wear and tear in archival collections, we found that such epidemiological studies can provide the framework to extract information from large populations, thus embracing their richness and diversity, which cannot be fully captured by experimental studies and models. Examples include the effect of the thickness of a stack of archival records on their wear and tear, or the effect of boxes to protect items from mechanical degradation, which had not been supported by evidence until now.

The answer is in the objects themselves
This study, along with our Heritage Science series of papers on collections demography (see here, here and here), forms a meaningful whole, exploring the epidemiology of library and archival collections as well as developing demographic models for preservation scenario appraisals. The philosophy of synoptic decision making that our research enables is based on environmental management principles, with which management of cultural heritage resources has a lot in common.
The much needed evidence to inform preventive conservation decisions is hidden within real objects. We just need to use the appropriate tools to extract it.
Read Part I and Part II of this research on the Heritage Science website.
Comments