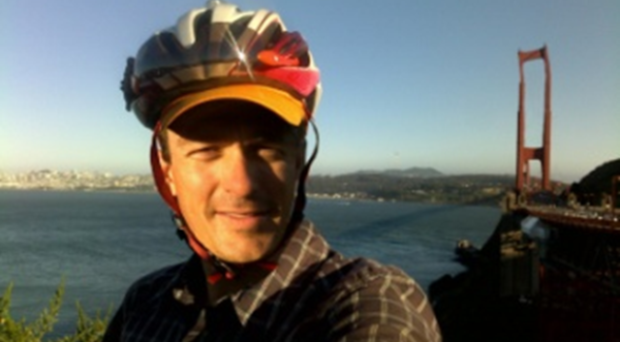
This blog was written by Stephan Lewandowsky and originally posted on the Psychonomic Society blog.
Earlier this month, the G20 summit brought the leaders of the world’s 20 most powerful nations to Hamburg, Germany, to discuss the issues facing our global society. The meeting was hosted by Angela Merkel, Germany’s chancellor, and among the guests were President Donald Trump and his Russian counterpart Vladimir Putin.
The New Statesman reported the excitement thus: “As the Russian president sat down for his long-awaited meeting with Donald Trump … the world held its breath. No one could look away. And nowhere was Putin’s pull better illustrated than in this viral photo of the leader, fixed with the rapt, almost adoring, gazes of Trump, Turkish president Recep Tayyip Erdogan, and Turkey’s foreign minister Mevlut Cavusoglu.”
Here is the picture:

It’s notoriously difficult to ascertain how many people have been exposed to viral content, but this picture did reach a lot of people: The search string “Putin picture G20 viral” returns nearly a million hits, and it appears that the picture originated with a Facebook post by a Russian journalist.
There is only one small problem: The picture was fake. Putin never sat in that chair which actually belonged to the British Prime Minister.
Social media quickly caught on:

Doctoring of images has become possible—and easy—through advances in digital technology. It also seems widespread and often involves people who should know better, namely professional photographers. Nearly 20% of finalists during a recent World Press Photo competition were disqualified because the entrants unduly enhanced the appeal of their photos during processing. And in 2015, the First Prize winner of the World Press Photo competition was stripped of the honor when irregularities with his submissions were detected.
So how can we tell whether an image is “fake” or “doctored”? The World Press Photo judges had access to the original raw files (i.e., as they were created by the camera when the shot was taken) as well as the files submitted for evaluation, so their task was relatively easy.
But what about the public? Can we tell whether a photo is fake? And how would we do this? Given that it is estimated that more than 14,000,000 photos are uploaded to Facebook every hour, this question is of considerable practical importance.
A recent article in the Psychonomic Society’s journal Cognitive Research: Principles & Implications tackled those questions. Researchers Sophie Nightingale, Kimberley Wade, and Derrick Watson explored people’s ability to detect common types of image manipulations by presenting them with a single picture at a time.
The researchers studied two types of image manipulations: Implausible manipulations might involve an outdoor scene with shadows running in two different ways (implying that there was not just a single sun in the sky), whereas plausible manipulations involved things such as airbrushing (e.g., of wrinkles) and additions (e.g., inserting a boat into a river scene) or subtractions (e.g., removing parts of a building) from the picture.
The figure below shows a sample stimulus with all of the various manipulations being applied. Specifically, panel (a) shows the original. The next two panels show plausible manipulations: In panel (b) sweat on the nose, cheeks and chin, as well as wrinkles around the eyes are airbrushed out. In panel (c) two links between the columns of the tower of the suspension bridge are removed. The remaining panels involve implausible manipulations: In panel (d) the top of the bridge is sheered at an angle inconsistent with the rest of the bridge, and in panel (e) the face is flipped horizontally so that the light is on the wrong side of the face compared with lighting in the rest of the scene. The last panel, (f) contains a combination of all those manipulations.

A large sample of online participants were presented with a series of such photos. For each photo, participants were first asked “Do you think this photograph has been digitally altered?” There were three response options: (a) “Yes, and I can see exactly where the digital alteration has been made”; (b) “Yes, but I cannot see specifically what has been digitally altered”; or (c) “No.”
If people responded with one of the “yes” options, they were asked to locate the manipulation. The same photo was shown again with a 3×3 grid overlaid and participants were asked to select the box that contained the digitally altered area of the photograph.
The results are shown in the figure below.

The light gray bars show people’s detection performance, which must be compared to the horizontal dashed line representing chance performance. (Chance here is at 50% because the two yes responses are considered together as one response option.)
It is clear that people were able to detect implausible image manipulations with above-chance accuracy. Performance increases even further when all possible image manipulations are combined. However, when an image is manipulated by airbrushing, people are unable to detect this alteration. Even a subtle addition or subtraction fails to elicit strong detection performance.
The dark gray bars show people’s ability to locate the alteration provided they had indicated its presence. The location performance must be compared against the white dashed lines which indicate chance performance for each type of alteration separately. (Chance differs across manipulation type.)
It is unsurprising that people could not locate the airbrushed alterations with above-chance accuracy, given that they had difficulty detecting the manipulation in the first place. What is perhaps more surprising is that people cannot always locate the alteration even if they are able to detect it. For the people that answered “yes” to the detection question, only 45% of manipulations overall could be correctly located in the picture.
A further experiment conducted by Nightingale and colleagues largely corroborated these findings. An interesting further aspect of the results across both experiments was that the likelihood of a photo being correctly identified as manipulated was associated with the extent to which the manipulation disrupted the underlying structure of the pixels in the original image. That is, Nightingale and colleagues found a correlation between detection performance and a digital metric of the difference between the original and the manipulated versions of each picture. The manipulations that created the most change in the underlying pixel values of the photo were most likely to be correctly classified as manipulated.
At first glance this result may appear entirely intuitive. However, the result is actually quite intriguing because participants never saw the same scene more than once. That is, no participant ever saw the non-manipulated version of any of the manipulated photos that they were shown. It follows that the extent to which the original photo was disrupted could be inferred from the manipulated version, which implies that participants were able to compare the manipulated photo with their expectations about what the scene “should” look like based on their prior experience with scenes and photos.
And when our prior experience tells us whom we would not expect to be surrounded by other world leaders, the image manipulation jumps out in an instant:

Comments