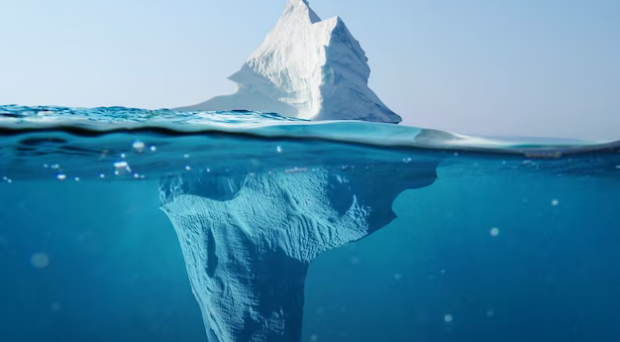
Randomised trials with a placebo control group are the gold standard for evaluating new treatments to improve health outcomes or to prevent disease. However, it may be unethical to randomise patients to placebo if there is already an established effective method for the treatment or prevention of the condition in question. In this case, a commonly-used design randomises patients to receive either the new treatment or the standard treatment (known as active-control trials). The aim of such trials is often an attempt to show that a new treatment retains most of the effectiveness of the standard treatment while offering other benefits in cost, simplicity, or side effects. We illustrate the problem with a hypothetical COVID-19 vaccine trial.
COVID-19 vaccine trial
The first licensed COVID-19 vaccine, BNT162b2 (BioNTech/Pfizer), was found to reduce the incidence of COVID-19 by approximately 95%. Imagine that we wished to assess a new COVID-19 vaccine after BNT162b2 had been licensed. Given the very high efficacy of BNT162b2, we decide to conduct a trial comparing the new vaccine versus BNT162b2. It is a large trial, with 10,000 persons followed for one year.
Imagine that the rate of COVID-19 cases in the trial population would have been 4% per year in the absence of vaccination and that the new vaccine is 80% effective. With these assumptions we would observe 20 COVID-19 cases in the BNT162b2 group and 80 COVID-19 cases in the experimental vaccine group (see Table below).
BNT162b2 | Experimental vaccine | Ratio (95% CI) | |
Person-years follow-up per arm | 10,000 | 10,000 | |
COVID-19 cases if subjects had not been vaccinated | 400 | 400 | |
Efficacy | 95% | 80% | |
Observed COVID-19 cases | 20 | 80 | 4.00 (2.42-6.90) |
Averted COVID-19 cases | 380 | 320 | 0.842 (0.794-0.894) |
Table. Values in bold are known or directly observed, other values are inferred.
The standard approach to analysing this hypothetical trial is to calculate the ratio of the numbers of observed cases in the two groups. Here, the value is 4, indicating that the new vaccine increases the risk of getting COVID-19 by a factor of 4, compared with BNT162b2. At face value, this analysis argues strongly against approving or using the new vaccine.
But this would mean rejecting a vaccine that we have assumed is 80% effective, much higher than the target of 50% that was set by the World Health Organization. 80% also happens to be the approximate efficacy of the ChAdOx1 viral-vector vaccine which is considerably cheaper than BNT162b2 and has less stringent cold chain requirements, a key consideration in resource-limited settings. Indeed, ChAdOx1 saved more lives worldwide in 2021 than any other COVID-19 vaccine. How do we resolve this paradox?
The importance of averted events
In our paper we argue that the interpretation of active-control trials must consider averted events as well as observed events. From Table 1 we see that BNT162b2 has averted 380 COVID-19 cases and that the new vaccine has averted 320 cases. The ratio of the numbers of averted cases is 320/380 = 0.842. The interpretation is that the new vaccine averts 84.2% of the COVID-19 cases that would have been averted by use of BNT162b2 (we call this the averted events ratio). This – rather than the ratio of 4 in terms of observed events – is the most relevant measure for policy makers trying to decide which vaccine to implement.
Naturally, if everything else was equal (including cost) then BNT162b2 would be the vaccine of choice, but real-world decisions are more complex than this.
Challenges
So far, we have skipped over a big problem in estimating the numbers of averted events: we need to make an assumption about the rate of COVID-19 in the absence of vaccination (we assumed 4% per year in our example). Without a placebo group, we cannot get this information directly from the trial and have to use external information instead.
Our paper discusses various approaches to address this problem. None of these are entirely satisfactory but we argue that this must be attempted to draw rational inferences. Our proposed method is especially relevant when the standard treatment is very effective, where it can lead to a substantial saving in sample size.
Comments