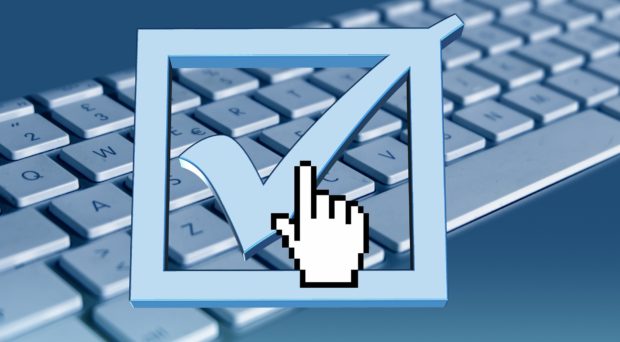
Despite occasionally coming under fire, pre-publication peer review remains the primary method of quality control in scholarly publishing.
However, peer review is a human system, with all of a human’s fallibilities. It can be inconsistent, subjective, potentially biased, with mounting frustrations regarding the time it takes and the burden it imposes, both on the authors and the reviewers themselves.
But for all its limitations, it has served science well, with widely-held views from recent surveys that – while it may not be perfect, it is nonetheless far better than anything else we have been able to devise.
A reviewer’s subject knowledge and ability to put research findings into a wider context are invaluable; however, there are some things reviewers are simply not best placed to check.
A reviewer’s subject knowledge and ability to put research findings into a wider context are invaluable; however, there are some things reviewers are simply not best placed to check.
If an article is not reported in sufficient detail, peer reviewers are unable to make judgement calls as to the validity and reliability of the results. To address this, reporting guidelines like the CONSORT Statement have been created, detailing the minimum set of items an author needs to provide for a complete and transparent account of what was done and seen.
However, despite the CONSORT Statement being published nearly 20 years ago, there is evidence that reviewers often fail to detect important deficiencies in reporting of the methods and results of randomised trials. Most non-specialist peer reviewers, despite their best intentions, are not qualified to critique methods or statistical analyses effectively.
What if we removed the person?
This is what Timothy Houle, Wake Forest School of Medicine, and Chadwick Devoss, Next Digital Publishing, asked. If a person is not well suited to check the statistical and methodological reporting in a manuscript, perhaps a computer could?
Together they have created a piece of software called StatReviewer, which ‘looks for’ critical elements in submitted biomedical manuscripts. StatReviewer scans the document looking for key phrases to identify the structure according to standard IMRAD (Introduction, Methods, Results and Discussion) headings, and parses the manuscript into the relevant sections.
It then runs thousands of algorithms on each section, comparing them against the relevant reporting guidelines to see if the information has been reported, and evaluating the appropriate use and reporting of statistical tests and p-values.
The output of this analysis is a numbered list of ‘suggested improvements’, which is exactly analogous to a traditional peer reviewer’s report.
Does it work in the ‘real world’?
We have been working with Tim and Chad for the last couple of years on this and they have progressed the system to a truly impressive level of accuracy for published articles, particularly for randomized controlled trials. But how will it cope with the ‘real world’?
While formats vary between journals, published articles have far greater structure than pre-review manuscripts and typos and spelling mistakes are uncommon, all of which makes StatReviewer’s role easier. Therefore, we have set out to see how it copes with the original submissions.
The pilot
We have begun a pilot evaluation of the software for clinical trials submitted to four BioMed Central journals – Trials, Critical Care, BMC Medicine and Arthritis Research and Therapy.
All new submissions to these journals will be evaluated for inclusion in the pilot. On submissions, manuscripts will undergo the pre-submission and editor checks as standard; however, once the manuscript progresses to peer review, relevant articles will be identified and sent to StatReviewer in addition to the normal peer review process of the journal. This additional review will in no way delay or interfere with the normal peer review process for the article.
Once all the reports have been collated, they will be sent to the handling editor and authors, with the StatReviewer report – clearly flagged as an automated review.
Our primary outcome for this pilot is simply to find out whether or not it works. We will be evaluating the StatReviewer report to see if it correctly identified all the missing and reported information correctly. We will also be comparing this to the (anonymized) ‘human’ reviews, to see if this represents an improvement over the current situation for these aspects.
One of the most interesting questions this poses is how authors will respond to an automated review.
One of the most interesting questions this poses is how authors will respond to an automated review. Will the fact that the review is computer-generated, and therefore the authors are unable to provide a rebuttal to the reviewer influence whether they are willing to follow its recommendations? For the sake of complete transparency, the StatReviewer report will be clearly marked as such, which also presents us with the opportunity to compare the authors’ responses to both the human and automated reviewers.
Going forward
This approach is not intended to replace the existing peer review process, but instead augment it in a similar way to how plagiarism software, such as CrossCheck, do. The ambition of StatReviewer is not to make judgement calls as to the quality of the science, but instead to provide a pre-review check of the reporting of a manuscript, providing prompt feedback to authors and ensuring reviewers have access to all the relevant information to make an informed decision.
Comments