This year’s International Society for Autism Research (INSAR) Annual Meeting is being held between 1st and 4th May. The meeting will be opened with a keynote address, ‘De novo Variation in Coding and Noncoding Regions: What We Can Learn from the Data About Etiological Pathways’ from Professor Kathryn Roeder.
Professor Roeder is Vice Provost for Faculty and Professor of Statistics and Computational Biology at Carnegie Mellon University. In addition to being the recipient of the 2013 Janet L. Norwood Award, and both the 1997 COPPS Snedecor and Presidents’ Award, she has published over 275 papers to date.
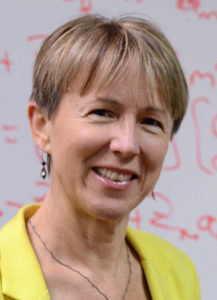
Thanks for taking the time to speak to us. Your research is predominantly based around statistical genetics. Would you be able to explain where your main fields of expertise lie, and how statistical genetics can be applied autism research?
KR: As a statistician, I naturally believe that statistical reasoning can be applied to almost any quantitative question. There are many open quantitative questions concerning autism research. My areas of expertise are statistical modeling, high-dimensional inference and machine learning.
While it is possible to find an off-the-shelf tool for many problems, I believe careful modeling is the first step to obtaining the optimal solution to nearly any statistical problem. In my opinion, the solution is optimal if it satisfies three constraints: 1) the model approximates the key features of the biological process; 2) it provides for powerful inferences about the question under investigation; and 3) the method is robust to the inevitable violations of the modeling assumptions.
Most genetic problems involve a huge number of variables: for example, millions of single nucleotide polymorphisms (SNPs) in the genome and tens of thousands of genes. Solving these problems requires tools of high-dimensional inference; the most familiar of these is principal component analysis, but other more flexible tools can provide improved inferences. Machine learning tools are ideal for the high-dimensional problems that arise in genetic research.
Finally, good statistical work is always paired with a good understanding of the scientific question under investigation. Much of my work is done in collaboration with Dr. Bernie Devlin, University of Pittsburgh School of Medicine. Bernie is expert in genetics and statistics and provides a bridge between the methods and the application.
Could you tell us about the themes of your keynote address at INSAR’s 2019 Annual Meeting?
KR: De novo variants have provided a powerful pathway to discover risk factors and genes involved in autism spectrum disorder (ASD). Most recently, the Autism Sequencing Consortium posted a manuscript in which we identified 102 risk genes, some new and others with stronger evidence than previously documented. It is exciting to have access to this substantial list of risk genes, but on their own, a list of genes does not provide much insight into the etiology of ASD. Thus, we explore several avenues to understand how, where and when these genes and mutations are likely to exert the most impact.
Single-cell and bulk RNA sequence data provide insights into which cell types are most impacted by mutation in ASD risk genes. Co-expression networks identify clusters of co-expressed risk genes, which implicate immature neurons in risk and link the transition from neural progenitors to neurons as one potential origin of atypical neurodevelopment in ASD.
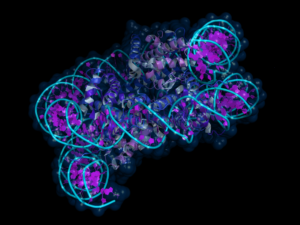
Alternatively, evaluation of de novo mutations that disrupt protein interactions provide insights at the protein level, revealing which gene-gene interactions have been disrupted and identifying hub genes in the disrupted interaction network. A substantial challenge we face is how to coordinate insights obtained from transcription with our findings from the protein domain. Indeed, while we often investigate gene interactions via co-expression, it is at the protein level that gene products usually interact. And yet protein data are not available for particular developmental periods or regions of the body, limiting its usefulness in our quest to understand the spatial-temporal dynamics. In the keynote address I’ll attempt to merge insights from transcription and protein interaction networks to gain greater understanding of the etiology of ASD.
I’ll also briefly touch on an exciting new area, namely how whole-genome sequencing results suggest that de novo mutations in promoter regions, characterized by evolutionary and functional signatures, contribute to ASD.
What are you most looking forward to seeing at INSAR this year?
KR: My work is focused on discovering risk genes, as well as their mechanisms of action, so I’m always most interested in what can be done in practice with these discoveries. Sessions that caught my eye are “Novel Therapeutic Approaches (gene, protein or RNA targeted therapies)” and “Drug Discovery and Development in ASD”.
You’ve published three articles in Molecular Autism in the past. Can you give us some background on one of these, and tell us why the findings are of importance to the field?
KR: Let me tell you about the 2012 Klei et al. article, because it relates to the work I’ll present in the INSAR panel session: ‘Molecular Genetics: Genetic and Genomic Discovery in Autism: From SNPs, to Exomes and Genomes’.
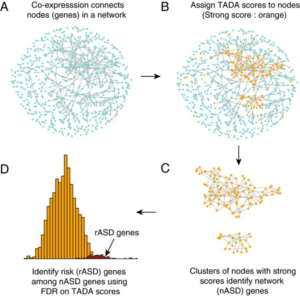
In that paper we obtained the best estimate of the heritability of autism attributable to common variants at that point in time. Previous estimates had underestimated this heritability because the studies used family-based samples instead of unrelated controls in their estimates. We later built on this finding in the Gaugler et al (2014) article, where we showed that most of the heritability of ASD is explained by common variants of small effect.
In the INSAR panel session I’ll take this line of research further still to show that while rare variation confers risk, both rare de novo and inherited variation acts within the context of a common-variant genetic load, and this load accounts for the largest portion of ASD liability. Even among individuals who carry rare highly damaging variants, there is sound evidence for substantial common risk variation, a result that is contrary to some popular beliefs.
If you enjoyed Professor Roeder’s keynote address and Q&A, you can read her articles in Molecular Autism by following the links below the blog.
Amy Joint
She studied Zoology at the University of Bristol, and in a previous life was a Stage Manager.
Latest posts by Amy Joint (see all)
- What makes a prize-winning paper? Observations from the 2020 Brain Structure and Function Editors’ Choice Award - 30th November 2020
- Brain Awareness Week 2020: Cognitive function and commercial video games - 16th March 2020
- Q&A with Professor Kathryn Roeder - 30th April 2019
Comments