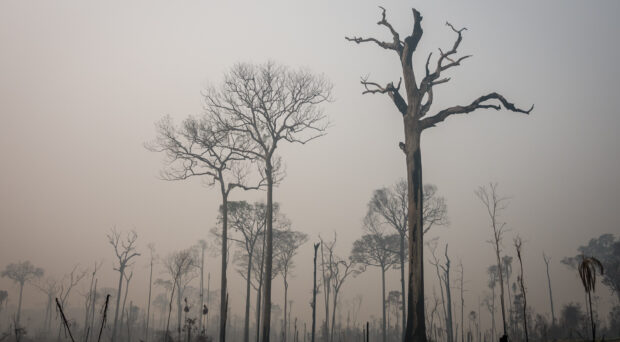
Last Saturday, 22 April 2023, was Earth Day, an international annual event to recognize, celebrate and advocate for the protection of the environmental and ecosystems. Perhaps due to the clever algorithms used by social media and search engine feeds I found myself reading through a really interesting paper on using socioeconomic and ecosystem degradation variables to improve the predictive accuracy of disease risk models for NTDs in Brazil.
Of Methods and Models
The researchers used disease occurrence data from the Brazilian Ministry of Health database (DATASUS), collecting infectious disease data from 2007 to 2019 using only laboratory confirmed incidence cases. For the infectious disease to be included in the modelling, it had to meet certain criteria; it had at least one species of vector, host or reservoir, and therefore, is influenced by environmental factors. They avoided high-coverage vaccine-preventable diseases such as yellow fever. The nine diseases that met these criteria were:
- Dengue Fever
- Malaria
- Chaga Disease
- Cutaneous Leishmaniasis
- Visceral Leishmaniasis
- Brazilian spotted fever
- Schistosomiasis
- Leptospirosis
- Hantavirus pulmonary syndrome
Exact location information was not available, so the authors used the municipality data and then standardized occurrences inside an 18km grid. One presence point per 18km cell was used.
Choosing predictor variables for models
The researchers collected bioclimatic variables using temperature and precipitation data, landscape change variables including natural habitat cover 2016 (sum of forest and savannah formation from each municipality), ecosystem destruction (2009-2018 sum of natural forest and grassland cover that were transformed into urban, pasture or plantation areas), socioeconomic variables: percentage households with piped water, percentage household without toilets, population density, mean human development index, Gini index from IBGE (a measure of income disparity), and per capita gross domestic product (GDP).
They used Ecological Niche Modelling for disease risk mapping: Two sets of models were compared to test whether socioeconomic variables improved the model’s performance for each disease: a set of models with environmental and socioeconomic variables (combined model) and a set of models with only environmental variables (simple model).
After checking for a correlation between predictor variables (highly correlated predictor variables can weaken models) the following were used for each set of models:
- Combined (socioeconomic and environmental):
- Mean annual temperature.
- Annual precipitation.
- Ecosystem destruction between 2009-2018.
- GDP.
- Gini coefficient.
- Proportion of households without a toilet.
- Simple (environment-only)
- Mean annual temperature.
- Annual precipitation.
- Ecosystem destruction between 2009-2018.
The researchers compared the quality and predictive performance of the two sets of models (combined and simple) for each disease and used them to identify which variables are most important in terms of their influence on the likelihood of disease occurrence for each disease.
Poverty and Ecosystem destruction as predictors of NTDs
The combined models that incorporated socioeconomic predictor variables with environmental variables overall performed 10% better than the simple models. However, the findings showed that this varied across diseases with the combined models performing significantly better for dengue fever, malaria, cutaneous leishmaniasis and leptospirosis, giving better defined disease risk areas, whereas the simple models performed better for Brazilian spotted fever.
Poverty and diseases: In the combined models, GDP was the most significant predictive variable for all diseases, followed by annual precipitation and annual mean temperature. Out of all the socioeconomic variables GDP was the most important variable for schistosomiasis, leptospirosis, malaria, dengue fever, visceral and cutaneous leishmaniasis and Chagas disease. For hantavirus and Brazilian spotted fever the most important socioeconomic variable was the proportion of households without toilets. Interestingly the authors noted that whilst for most diseases lower-income and poverty was associated with increased likelihood of disease, this was not the case for dengue fever where higher income municipalities had higher risk of dengue fever. The authors suggest that this may be because dengue transmission can be better sustained in urban areas.
The authors discussed this relationship between poverty and the increased likelihood of disease and the poverty trap process, which locks communities into cycles of disease and poverty that spans generations, and argue that a combination of public health and social policies are need to reduce poverty, address disease transmission risk and break the poverty trap.
Ecosystem destruction and disease risk: In the simple models the most important environmental variable was ecosystem destruction (or land cover change) for leptospirosis, cutaneous leishmaniasis, dengue fever, schistosomiasis, hantavirus and Chagas disease. The researchers found that this relationship between ecosystem destruction and increased disease likelihood showed a negative downward trend. The researchers concluded this meant that infection transmission risk increased for these disease during the early stages of ecosystem degradation, e.g. when pristine forests or savannas are destroyed through deforestation or digging, As a result, the parasite transmission cycles in the area also change, potentially leading to disease emergence, spillover events (e.g. when a parasite that normally infects wildlife starts infecting humans), or increased human contact with transmission sites. If the ecosystem degradation is historic and there is no ongoing landscape change then the transmission patterns may be stable, with little spillover or disease emergence events.
The authors highlight how these findings support the argument that poverty and ecosystem destruction increases disease transmission risk, and that human population health security can not be assured without addressing ecosystem health. This is supported by the One Health concept that seeks to protect and improve the health of people, other animals, and the environment. This can be achieved by using a multisector/multi-disciplinary coordinated approach to identify, monitor and reduce health threats at the human-animal-ecosystem interface.
Comments