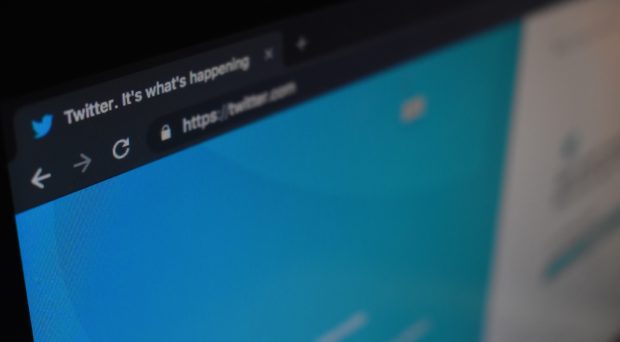
This blog has been cross-posted from the SpringerOpen blog
Despite the many scandals surrounding social media companies and their practices of data sharing, they are still central platforms of opinion formation and public discourse. Therefore, social media data is widely analyzed in academic and applied social research. Twitter has become the de facto core data supplier for computational social science as the company provides access to its data for researchers via several interfaces. One of these – the “Sample API” – is promoted by Twitter as follows:
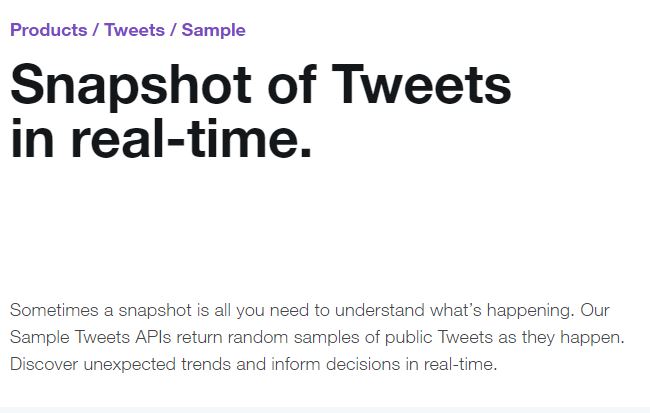
Twitter’s Sample API provides 1% of all Tweets worldwide for free, in real-time – a great data source for researchers, journalists, consultants and government analysts to study human behavior. Twitter promises “random” samples of their data. The randomness of a sample – each element has an equal probability of being chosen – is of high importance for social scientific methodological integrity as a sample selected randomly is regarded as valid representation of the total population. Even though Twitter shares (parts of its) data with potentially everybody (unlike other social media companies), the company does not reveal details about its data sampling mechanisms.
Even though Twitter shares data with potentially everybody, the company does not reveal details about its data sampling mechanisms
We set up experiments to test the sampling procedure of the Sample API by inducing tweets into the feed in such a way that they appear in the sample with high certainty. In other words, while a Tweet should have a 1% chance to be part of the Twitter’s 1% sample data, it is easily possible to increase that chance to 80%. Consequently, finding 100 Tweets in the 1% sample related to a certain topic might not result from a random sample of 10,000 Tweets but just from a manipulated sample based on 125 Tweets.
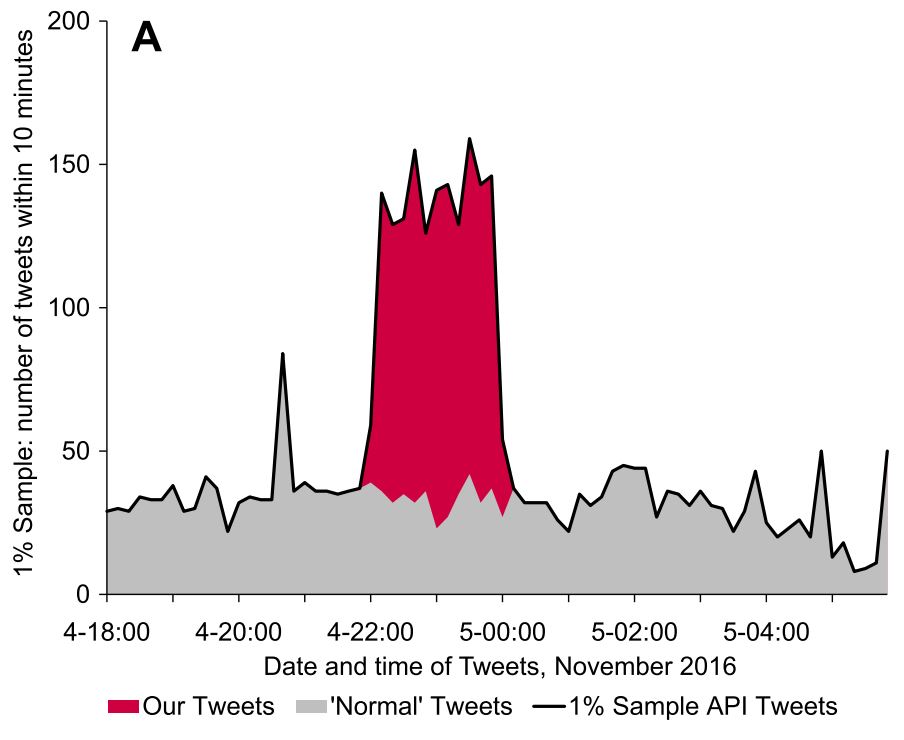
This figure illustrates the effect of a Tweet injection experiment during the Nov 2016 US presidential election campaigns using the hashtag #trump. The gray area represents Tweets in 1% sample from 328 million users, red represents the induced tweets, the black line illustrates the 1% Sample API Tweets. One hundred accounts were enough to manipulate the data stream for a globally important topic.
100 accounts were enough to manipulate the data stream for a globally important topic
We also developed methods to identify over-represented user accounts in Twitter’s sample data and show that intentional tampering is not the only way Twitter’s data can get skewed. For instance, automated bots can accidentally be over-represented in the data samples or be invisible at all. The authors also show evidence that corporate Twitter users seem to be allowed by Twitter to send many more Tweets than regular users, which will automatically inflate their position in the data.
Our study lists potential solutions both for the architectural flaws and the regaining of scientific integrity. The latter could be achieved by making sampling methods transparent and cooperating with social media researchers more closely to create open interfaces as well as the possibility to better assess the data at hand. At a time when decision making is based increasingly on the analysis of social data, also industry should do everything to enhance public trust in the methodologies at hand.
Our study lists potential solutions both for the architectural flaws and the regaining of scientific integrity
Even though some big data evangelists state that sampling is “an artefact of a period of information scarcity”, reality makes sampling a central necessity in times of information abundance. Researchers have to trust Twitter to supply them with methodologically sound samples while dealing with all kinds of other problems, such as bias and ethical issues (see here, here and here).
Comments