One of the essays in the fascinating – and open access – text on data-intensive science, The Fourth Paradigm, envisages an age of instantaneous knowledge translation, where scientific discovery can be instantly applied to clinical practice. This concept was named the ‘healthcare singularity’ and its achievement will involve real-time generation, integration and processing of human genetic and clinical disease data. The axis (pictured) is approaching but will remain elusive without fundamental changes to the way science is conducted, and communicated.
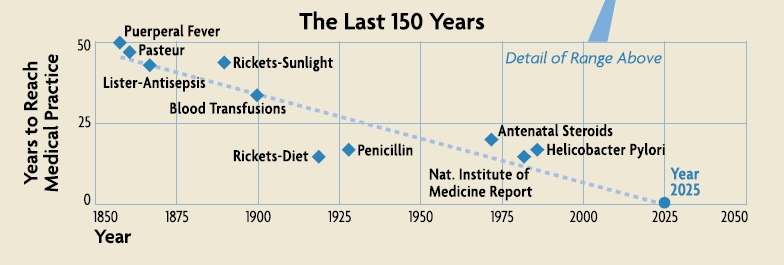
Image adapted from: Gillam et al.: The Healthcare Singularity and the Age of Semantic Medicine. In The Fourth Paradigm (2009)
Open access to scientific data is a means to achieve the healthcare singularity’s end – of more efficient, reliable and reproducible research which will ultimately improve human health. Dr Eric Schadt (Mount Sinai School of Medicine and Editor-in-Chief of BioMed Central journal Open Network Biology) and John Wilbanks (Senior Fellow at the Kauffman Foundation, Research Fellow at Lybba and Open Network Biology Editorial Board member) are two scientists working to transform research on human disease.
A major barrier to effective sharing of genetic and clinical data are suboptimal processes for obtaining informed consent from patients, and intellectual property restrictions placed on individual patient data obtained through research. One of the outcomes of Workgroup D at this spring’s Sage Commons Congress, attended by BioMed Central, was to develop a suite of legal tools to empower research participant control over the use and access to their samples and data. John Wilbanks – who recently left his full-time post as Vice President for Science at Creative Commons to focus on data sharing – is taking on this challenge with his latest project, Consent to Research, which celebrates its alpha release today.
And once we have more ready access to these complex, heterogeneous and voluminous data types – genotype, gene expression, clinical, and others – they need to be interpreted and put to effective use for fighting disease. Eric Schadt is no stranger to this kind of “extreme science” and recently joined Mount Sinai Medical School to further his study of complex human disease biology, and apply it to medical treatments.
Amongst their moves to new positions, occurring at each end of the summer, Eric and John have been leading and supporting, respectively, the launch of the aforementioned new BioMed Central journal, Open Network Biology. Here they answer some questions about their latest projects, the new journal, and how we might achieve more universal and effective personalized healthcare.
Eric Schadt – Q&A
Why do we need Open Network Biology, who is the journal for, and what are you doing differently?
Today one of the primary end results of research is a scientific
publication. Particular results from a study are presented by authors in a paper. Historically,
this was a great way to communicate “simple” results, based on single or
small sets of genes or proteins characterized in simple contexts.
Today, papers are rapidly evolving into advertisements of which vast amounts of data scored in populations are reduced to a few
straightforward results, leaving giga- or even terabytes of
underlying data and scores or hundreds of pages of supplementary
material for the reader to access if they so choose. Only a small
fraction of what is learned from these data is represented in the main
text. The data may be available but are usually difficult to assemble,
difficult for any reader to reproduce the results presented in the
paper – even if they have access to the code and data.
Open Network Biology seeks to
help drive a transformation in the
biological sciences wherein models of biology are one of the primary
end points of research. By publishing the
model, the data upon which the model is based, and the code used to
generate the model, and then providing a platform on which readers can
execute the code, readers and reviewers will not only be able directly
reproduce a given model, but then interact with it, test it, validate or
invalidate it. Open Network Biology will
also consider more classic types of manuscripts, like
methodological advances in constructing predictive models and networks,
commentary, and review articles highlighting the importance of
network-based models now relevant to a wide variety of disease areas such
as cancer, immunology, neuroscience, and infectious diseases. There
is now the ability to generate unprecedented scales of data in these fields.
What exactly is a network model of disease and what problems do/will they solve?
Networks are a convenient framework for organizing big data,
relating many variables to one another at a hierarchy of levels.
Fundamentally, a network is comprised of nodes and edges, where the
edges can be directed or undirected. In the biological context the
nodes typically represent RNAs, proteins, metabolites, cellular
phenotypes or higher order phenotypes associated with disease. Edges
between the nodes indicate a relationship between two nodes (see figure).
Figure. Network of gene expression and clinical traits previously identified as dramatically enriched for genes that are predicted as causal for obesity, diabetes and heart disease. The blown up subfigure indicates the type of causal connections that can be explored in a model to identify genes that serve as causal regulators for disease-associated networks. Red nodes indicate gene and clinical trait values that are down regulated and green is upregulated in the highlighted subnetwork.
A network could be very simple, even a single gene, where changes in that single node could give rise to disease (e.g., if you have a certain number of CAG repeats in the Huntington’s gene, you will develop Huntington’s Disease), or very complex, in which a significant proportion of the genes in the network give some risk of disease (as in the Figure), so that the overall state of the network must be understood in order to accurately predict disease risk. If you have a reasonably accurate network of disease it solves many problems. For example, you can identify the best nodes to assay to assess the state of the network, the best nodes to track to assess disease risk or progression, and the best nodes in the network to target for therapeutic intervention.
Nearly everyone who’s online today is part of social network – are there any similarities with biological networks?
Absolutely many similarities and at many different levels. For example, many social networks have been shown to be scale free, where a smaller percentage of nodes are connected to many other nodes, and then most other nodes are connected to a smaller number of nodes. Protein interaction networks and gene expression networks have been shown to follow a similar distribution. Interestingly, recent work has also shown that the structure of the social network in which you are part may define your risk of disease. For example, those individuals who are in closer proximity to individuals with obesity, tend to be at increased risk of developing obesity, not unlike increased risks of infection or other communicable diseases. At some point we will want to study networks at a hierarchy of levels, at multiple scales, beginning with molecular networks and how they interact with cellular networks, and how these interact with tissue or organ based networks, on up to organisms, and finally up to entire communities.
The National Health Service in the UK was recently reported to be “unprepared” for genomics – how, in your view, do we make universal personalized healthcare a reality?
I think in this context by wholly unprepared the meaning may have been from the standpoint of healthcare providers generating whole genome sequencing data, storing it, managing and organizing it, integrating it into a patient’s healthcare record, enabling the physician to interact with the data so as to make informed decisions regarding diagnosing and treating a patient. This is a daunting task and presently only a few high-end research centers on the planet have mastered the ability to generate, organize, and compute on these scales of data (the raw data from a sequencing run on a single sample can be from 1-5 terabytes!). It will take time to capture what it takes those centers to master and repackage it in a way that can be taken up by others who are not as information savvy. For sure there are many and a growing number of examples where routine sequencing of panels of genes can impact clinical decision making. But for most forms of common human diseases this is not the case. Only by integrating many different types of data (DNA, RNA, proteomic, metabolomics, clinical) to construct predictive models of disease will we achieve a more complete understanding of disease.
What motivated your move to New York?
I moved to New York to fully engage the type of cutting edge technology Pacific Biosciences has developed with medicine. The opportunity at Mount Sinai School of Medicine in New York was to help transform the practice of medicine by generating the right scales of data. We can leverage the large patient population at Mt. Sinai, the electronic medical records, and pull in all of the available data from the public domain – the literature, the big stores of genomic data – and then integrate these data, build better models of disease, and enhance physicians’ ability to make decisions. So really very exciting.
Finally, what needs to change in research and scholarly communication to achieve the healthcare singularity?
As a research community, if we want to move beyond our current state, if we really want to achieve something like the healthcare singularity, then we need to find a better way to persist the vast stores of knowledge that obtain from models built upon vast stores of biological data. Only by making knowledge derived from big data accessible can we engage the research community, clinicians and even patients to achieve wisdom regarding the causes and best ways to prevent and treat disease. Importantly, we need to change the culture, the way in which we generate big data sets, the incentive structure, and the way we communicate research results if we want to get to the healthcare singularity more quickly. Today government funding agencies largely fund individual groups or small numbers of groups to carry out studies, generate data, and carry out focused experiments to increase our understanding of disease. This I believe is grossly inefficient, highly redundant and ultimately under powers the individual researcher to really understand complex living systems. In areas like high-energy particle physics, there are a few centers funded to build the big equipment, generate the big data, and then those data are more openly shared with the broader community for all to explore. We need to move in that direction in the biomedical and life sciences as well. We need to be looking at entire populations and doing it over time, scoring many different traits at a hierarchy of levels, if we want to really achieve understanding. This is not within the capability of any single lab, any single university, any single company, but instead must be done more collectively. This of course will require a more fundamental shift in the current culture in the biological sciences. Being really open with data, sharing all data as soon as it is generated, is not how we typically operate in the biological sciences, although genomics, the sequencing of the human genome, demonstrates that it is certainly possible to generate big data and immediately share that data with the community at large. Those who are funded by government agencies, with money from tax payers, should not be allowed to hoard the data, to “own” it and control it as this only slows down our ability to achieve understanding from the data. The data should be seen as being owned by the people, not by the individual investigator who is funded to generate the data. Of course, this will then demand changes in how universities reward faculty, how a faculty gets tenure, how researchers are incentivized to take on specific projects. Being a first or last author on many papers as a key metric of your worth as an independent investigator, must give way to other metrics that can assess what your contribution has been to understanding different models of biology.
John Wilbanks – Q&A
Why do we need the Consent to Research project?
Because the way we do informed consent right now is simply not keeping up with technology. We built the informed consent system before the Internet. It wasn’t designed for a world of big data, and so it doesn’t facilitate the use of big data. It keeps data fragmented from a legal perspective through the use of a unique agreement for every research project, and that’s holding us back. Research projects often gather the same data (which means we’re wasting time and money redoing work that’s already been done) or gather data that is connectable to other data (which means we’re wasting opportunities to make new discoveries and connections). Consent to Research is creating standardized informed consent agreements. They’re completely voluntary, they’re technically enabled, and they’re designed from the ground up to connect research, not fragment it. They build a commons of data for research, a commons that is user-generated and directly available to researchers who agree to a certain set of norms: to play fair and to publish openly. We’re standing on the shoulders of giants. There’s a lot of good in that informed consent system, and we’re leveraging that. But it also is bringing some of the lessons we’ve learned in technical standards and the emergence of public legal tools like the Creative Commons copyright licenses – that having at least some standards can enable enormous innovation, that user interfaces to complex legal agreements are incredibly powerful, and that conditional transactions (I give you something if you agree to behave a certain way) can be the thin edge of the wedge that move closed systems towards open ones.
Image credit Joi Ito: available under a CC-BY license
Who, in your view, really owns genetic and clinical data?
This is a very complex question. “Ownership” is an idea created by the law. We can own physical property because the governments under which we live say we can. It’s the same with copyrights, or with patents – they create these social contracts that say we own a book we wrote, or an invention we came up with. But data doesn’t have that kind of social contract over it automatically, so there’s not a clear answer to who “owns” it. The reality is that as long as you’re the only person with your data, you “own” it in the sense that it’s up to you to decide who you give it to. But we increasingly live in a world in which our devices, especially our computers, tablets and phones, harvest “our” data and turn it over to companies who make sure they own the copy they get. They do this by imposing a contract on you, one you most likely never read because it’s 90+ pages long, in which you agree that they own your data. I prefer not to think in terms of ownership, but instead in terms of who has rights to your data and what rights they have. I like the idea that my data is somehow mine, but not in the sense of property, in the sense that I’m in charge of who is allowed to use it, and for what purpose. Because the property laws don’t fit the reality of data. If the data exist and are on the web, ownership is a pretty useless concept – but the idea of rights isn’t.
Studies have shown patients given more information about risks are less likely to agree to healthcare interventions – what does this mean for Consent to Research?
Not a whole lot. We’re not looking for people who get scared by getting more information – the traditional system serves them pretty well already. We’re looking for people who are willing to endure some friction as they go through a ten minute process that explains the Portable Consent framework we’re creating, who want the right to carry their consent around, to wear it as a badge of pride. We don’t need 20 million people, though of course that would be nice. We need people who want cures. Who want answers. Who want more engagement in health care, not less. That’s our target audience. And that’s why we’re working with groups in the disease advocacy community. They’ve got users who are motivated to take charge of their own care and their own diseases, and they’re willing to get their data out there for researchers to use.
How is Consent to Research different from existing patient data-centred initiatives, such as PatientsLikeMe, and THAT’S MY DATA?
These are two different projects. PatientsLikeMe is a business, and provides an incredibly rich experience for the patients who log in and use it to track their lives and health. We’re not trying to provide that kind of experience. But PatientsLikeMe relates to Consent to Research because they’ve pledged to give their users the power to download all their data under the Blue Button standard by Spring 2012 – and they’ll then be able to use Consent to Research to upload that data into a common repository where it can be correlated to their genomes and other information. Conversely, Consent to Research will also be able to harvest data from Consent to Research to make their patient experience even richer, which will hopefully start to create some awesome feedback loops between self-observation and genetics that have so far been hard to jump-start. THAT’S MY DATA is more closely tied to us. It’s another project emerging from the last Sage Congress (they were in the Group A working session, we were in Group D) and it’s based on the idea that if you give a biosample, you should get your raw data back. We’re specifically enabling that in our first Portable Consent agreement and will be creating more tools that enable it as we go along.
How will Consent to Research complement the goals of Open Network Biology, and Sage Bionetworks?
Our goal is to enable the emergence of a big, probably messy, batch of user generated data that can be processed into cleaner data and used in modeling. It’s that simple. Sage Bionetworks is going to be the first, but far from the last, organization to process, clean, and model with it. Hopefully that means more papers in Open Network Biology, more models in Open Network Biology, and in general that the field of modeling can start to scale faster than it is today. If scientists like Eric Schadt have to go collect all the data they analyze, that’s a rate limiting factor. If people like me can sign up and feed data to Eric automatically, then the models can start to grow a lot faster.
Finally, what needs to change in research and scholarly communication to achieve the healthcare singularity?
I don’t know what the healthcare singularity is, honestly. I just know that what we’re doing isn’t working. We need a lot to change – tenure and review systems in research, business models in scholarly research communication, funding policy and national and international levels, participation of scholarly societies, and more. But I tend to think that making a ton of data available without a lot of silos will serve as a strong attractor for the smartest people in the world to come and begin building things that utterly surprise and shock us. Being a futurist is ultimately about the present, not the actual future. I’m obsessed with creating environments that foment innovation, and bear the strong bias that the less we assume about what the outcomes will be and the more kinds of outcomes we enable, the more likely we are to achieve great progress.
Contact John to find out more about Consent to Research, and the Open Network Biology editorial office to discuss the new journal.
Comments